Event debrief: GWD Western Cape Talk on Machine Learning modelling techniques for groundwater availability in RSA Dolomites
Event debrief: GWD Western Cape Talk on Machine Learning modelling techniques for groundwater availability in RSA Dolomites
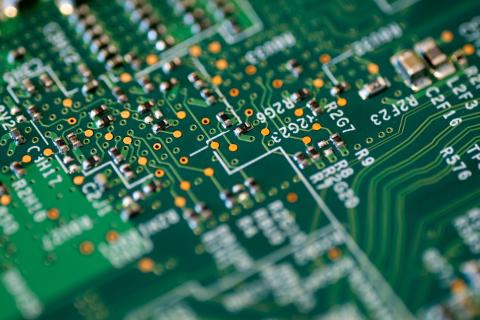
The GWD Western Cape Branch under the vibrant chairpersonship of Dr Sumaya Israel, started off 2021 with this interesting topic presented by the much esteemed Helen Seyler - Thank you so much for sharing your research results with us Helen!
This talk presented research into the applicability of machine learning for the forward prediction of groundwater levels and flow regime, as an alternative to numerical modelling, using results from the dolomite aquifers in South Africa. The research supports a larger programme researching the use of big data analytics for water secure transboundary systems.
Talk Abstract:
Fundamental to the definition of groundwater availability and the management of any aquifer is an understanding of the changes in groundwater levels and storage, recharge, and groundwater discharge to surface water, when the aquifer is pumped. This understanding forms the foundation for the determination of limits of future abstraction and thresholds of unacceptable impact, and provides a tool against which to compare future datasets and make groundwater management decisions.
Given the complex nature of groundwater and the interdependent responses of the system to change, quantifying the relationship between the aquifer flow regime and abstraction, and determining the long-term implications of different thresholds on these systems requires the use of models. Generating accurate simulations for groundwater behaviour with numerical models is however challenging due to the requirement to accurately understand the physical system in order to simulate it and overcome the non-uniqueness of the numerical solutions, which in turn requires detailed datasets. It has therefore become attractive to test the application of machine learning techniques in the simulation of groundwater behaviour.
About the speaker:
Helen has thirteen years experience as a hydrogeologist (in South Africa), including experience in various aspects of groundwater resources management, and specializing in numerical modelling for water resource quantification and scenario planning, wellfield operating rules, surface water – groundwater interactions, and the groundwater aspects for mining EIAs. She has a particular interest in "sustainable" groundwater use, and in social and economic development challenges as they relate to resources management. Her PhD thesis currently underway: Groundwater Decision Support Systems including Sustainability Indicators for Sustainable Groundwater Use.
Talk Resources
A recording of this Talk is available on the GWD YouTube Channel - only on request. Please email us for a link: [email protected]
Helen addressed these questions from participants during her session. Please refer to the Talk recording for her detailed feedback:
Arrey Agbor Q: Is the licensed abstraction data from the same area? And it would be interesting to hear how you dealt with the sources of errors with your data.
Nico van Zyl Q: Helen, why do you think LSTM Model performed better?
Sonia Veltman Q: Would you be able to overcome the storage effects with training the models with results from i.e. two layered numerical models? Where at first you calibrate the groundwater system through the numerical model and then use those time data sets to train the ANNs.?
Arrey Agbor Q: How about combining both ML and numerical models for explainability?
In addition, Helen shared an extract from the WRC report:
Tensorflow (Al-Abadi, 2016) in Python (Rossum, 1995) was used to model the LSTM (McKinney, 2010). Pandas, NumPy (Van Der Walt et al., 2011) and Matplotlib (Hunter, 2007) libraries were imported for management, processing and visualisation of data.
The nnetar function in the forecast package (Hyndman and Athanasopoulos, 2014) in R (R Core Team, 2017) is used to fit the NNAR model to the time series. The nnetar implements the NNAR model using a neural network with a sigmoid activation function, a single hidden layer and lagged inputs for forecasting the target variable.
Following this Talk, we are excited to announce the next GWD Western Cape speaker Zaheed Gaffoor will join us to present on the topic: "Localizing regional scale groundwater big data using machine learning: A case study of the Ramotswa/NW/Gauteng Dolomites" early March 2021.